Artificial intelligence: Making the black box of AI understandable, comprehensible and explainable with Explainable AI (XAI), heatmaps, surrogate models or other solutions
Published on: September 8, 2024 / Update from: September 9, 2024 - Author: Konrad Wolfenstein
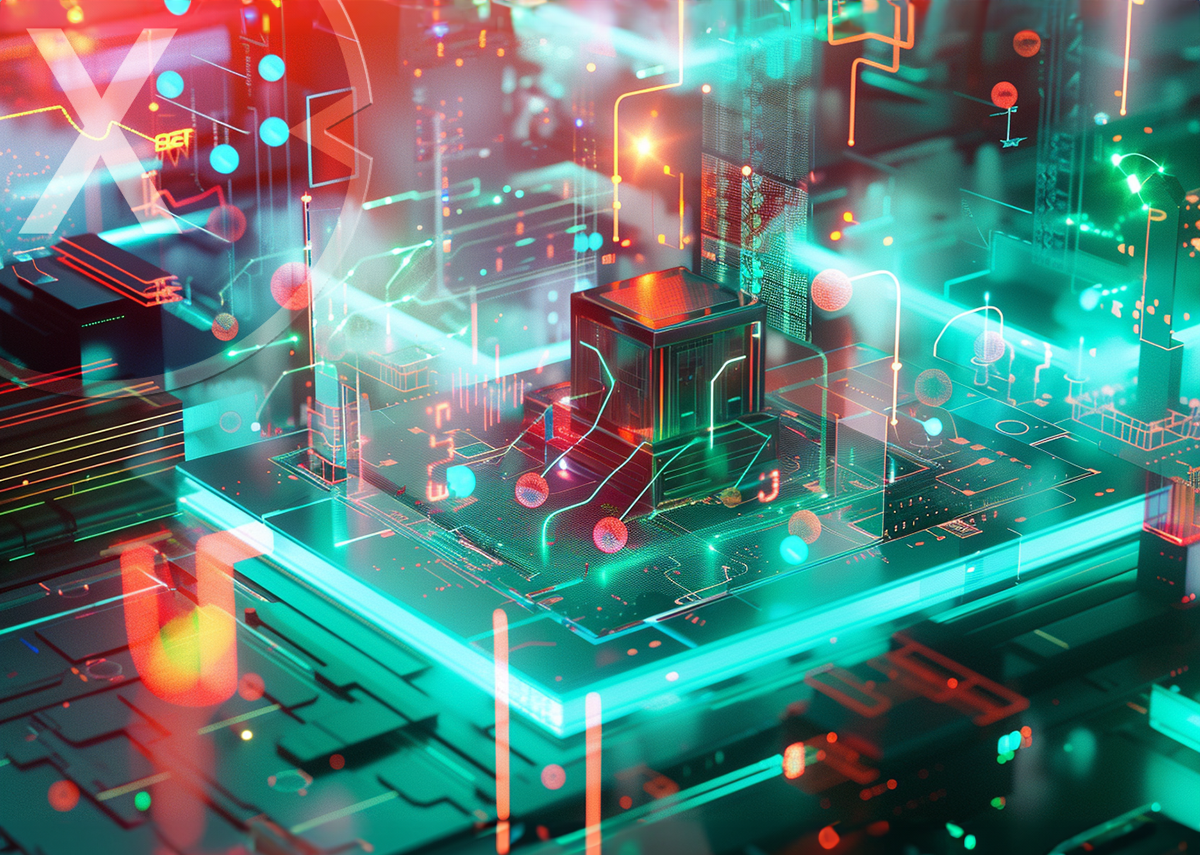
Artificial intelligence: Making the black box of AI understandable, comprehensible and explainable with Explainable AI (XAI), heatmaps, surrogate models or other solutions - Image: Xpert.Digital
🧠🕵️♂️ The puzzle of AI: The challenge of the black box
🕳️🧩 Black-Box AI: (Still) lack of transparency in modern technology
The so-called “black box” of artificial intelligence (AI) represents a significant and current problem. Even experts are often faced with the challenge of not being able to fully understand how AI systems arrive at their decisions. This lack of transparency can cause significant problems, particularly in critical areas such as economics, politics or medicine. A doctor or medical professional who relies on an AI system to diagnose and recommend therapy must have confidence in the decisions made. However, if an AI's decision-making is not sufficiently transparent, uncertainty and potentially a lack of trust arise - in situations where human lives could be at stake.
The challenge of transparency 🔍
To ensure the full acceptance and integrity of AI, a number of hurdles must be overcome. The AI's decision-making processes must be designed to be understandable and comprehensible for people. Currently, many AI systems, especially those that use machine learning and neural networks, are based on complex mathematical models that are difficult to understand for the layperson, but often also for experts. This leads to viewing the AI's decisions as a kind of "black box" - you see the result, but don't understand exactly how it came about.
The demand for explainability of AI systems is therefore becoming increasingly important. This means that AI models not only need to provide accurate predictions or recommendations, but should also be designed to reveal the underlying decision-making process in a way that humans can understand. This is often referred to as “Explainable AI” (XAI). The challenge here is that many of the most powerful models, such as deep neural networks, are inherently difficult to interpret. Nevertheless, there are already numerous approaches to improving the explainability of AI.
Approaches to explainability 🛠️
One of these approaches is the use of replacement models or so-called “surrogate models”. These models attempt to approximate how a complex AI system works through a simpler model that is easier to understand. For example, a complex neural network could be explained using a decision tree model, which is less precise but more understandable. Such methods allow users to get at least a rough idea of how the AI reached a particular decision.
In addition, there are increasing efforts to provide visual explanations, for example through so-called “heatmaps”, which show which input data had a particularly large influence on the AI’s decision. This type of visualization is particularly important in image processing, as it provides a clear explanation of which areas of the image the AI paid particular attention to in order to reach a decision. Such approaches help increase the trustworthiness and transparency of AI systems.
Important areas of application 📄
The explainability of AI is of great relevance not only for individual industries, but also for regulatory authorities. Companies are dependent on their AI systems not only working efficiently, but also working legally and ethically. This requires complete documentation of decisions, especially in sensitive areas such as finance or healthcare. Regulators such as the European Union have already begun to develop strict regulations on the use of AI, particularly when used in safety-critical applications.
An example of such regulatory efforts is the EU AI regulation presented in April 2021. This aims to regulate the use of AI systems, especially in high-risk areas. Companies that use AI must ensure that their systems are traceable, secure and free of discrimination. Especially in this context, explainability plays a crucial role. Only if an AI decision can be transparently understood can potential discrimination or errors be identified and corrected at an early stage.
Acceptance in society 🌍
Transparency is also a key factor for the broad acceptance of AI systems in society. To increase acceptance, people's trust in these technologies must be increased. This applies not only to professionals, but also to the general public, who are often skeptical about new technologies. Incidents in which AI systems made discriminatory or erroneous decisions have shaken many people's trust. A well-known example of this is algorithms that were trained on distorted data sets and subsequently reproduced systematic biases.
Science has shown that when people understand the decision-making process, they are more willing to accept a decision, even if it is negative for them. This also applies to AI systems. When the functionality of AI is explained and made understandable, people are more likely to trust and accept it. However, a lack of transparency creates a gap between those developing AI systems and those affected by their decisions.
The future of AI explainability 🚀
The need to make AI systems more transparent and understandable will continue to increase in the coming years. As AI continues to spread into more and more areas of life, it will become essential that companies and governments are able to explain the decisions made by their AI systems. This is not only a question of acceptance, but also of legal and ethical responsibility.
Another promising approach is the combination of humans and machines. Instead of relying entirely on AI, a hybrid system in which human experts work closely with AI algorithms could improve transparency and explainability. In such a system, humans could check the AI's decisions and, if necessary, intervene if there are doubts about the correctness of the decision.
The “black box” problem of AI must be overcome ⚙️
💡 Explainable AI: Ways out of opacity
📣 Similar topics
- 🤖 “Black box” of artificial intelligence: A deep problem
- 🌐 Transparency in AI decisions: Why it matters
- 💡 Explainable AI: Ways out of opacity
- 📊 Approaches to improve AI explainability
- 🛠️ Surrogate models: A step towards explainable AI
- 🗺️ Heatmaps: Visualization of AI decisions
- 📉 Important application areas of explainable AI
- 📜 EU Regulation: Regulations for high-risk AI
- 🌍 Social acceptance through transparent AI
- 🤝 Future of AI explainability: Human-machine collaboration
#️⃣ Hashtags: #ArtificialIntelligence #ExplainableAI #Transparency #Regulation #Society
🧠📚 An attempt to explain AI: How does artificial intelligence work and function - how is it trained?
An attempt to explain AI: How does artificial intelligence work and how is it trained? – Image: Xpert.Digital
How artificial intelligence (AI) works can be divided into several clearly defined steps. Each of these steps is critical to the end result that AI delivers. The process begins with data entry and ends with model prediction and possible feedback or further training rounds. These phases describe the process that almost all AI models go through, regardless of whether they are simple sets of rules or highly complex neural networks.
More about it here:
We are there for you - advice - planning - implementation - project management
☑️ SME support in strategy, consulting, planning and implementation
☑️ Creation or realignment of the digital strategy and digitalization
☑️ Expansion and optimization of international sales processes
☑️ Global & Digital B2B trading platforms
☑️ Pioneer Business Development
I would be happy to serve as your personal advisor.
You can contact me by filling out the contact form below or simply call me on +49 89 89 674 804 (Munich) .
I'm looking forward to our joint project.
Xpert.Digital - Konrad Wolfenstein
Xpert.Digital is a hub for industry with a focus on digitalization, mechanical engineering, logistics/intralogistics and photovoltaics.
With our 360° business development solution, we support well-known companies from new business to after sales.
Market intelligence, smarketing, marketing automation, content development, PR, mail campaigns, personalized social media and lead nurturing are part of our digital tools.
You can find out more at: www.xpert.digital - www.xpert.solar - www.xpert.plus