Data is the crucial component for generative AI - About the importance of data for AI
Published on: August 12, 2024 / Update from: August 12, 2024 - Author: Konrad Wolfenstein
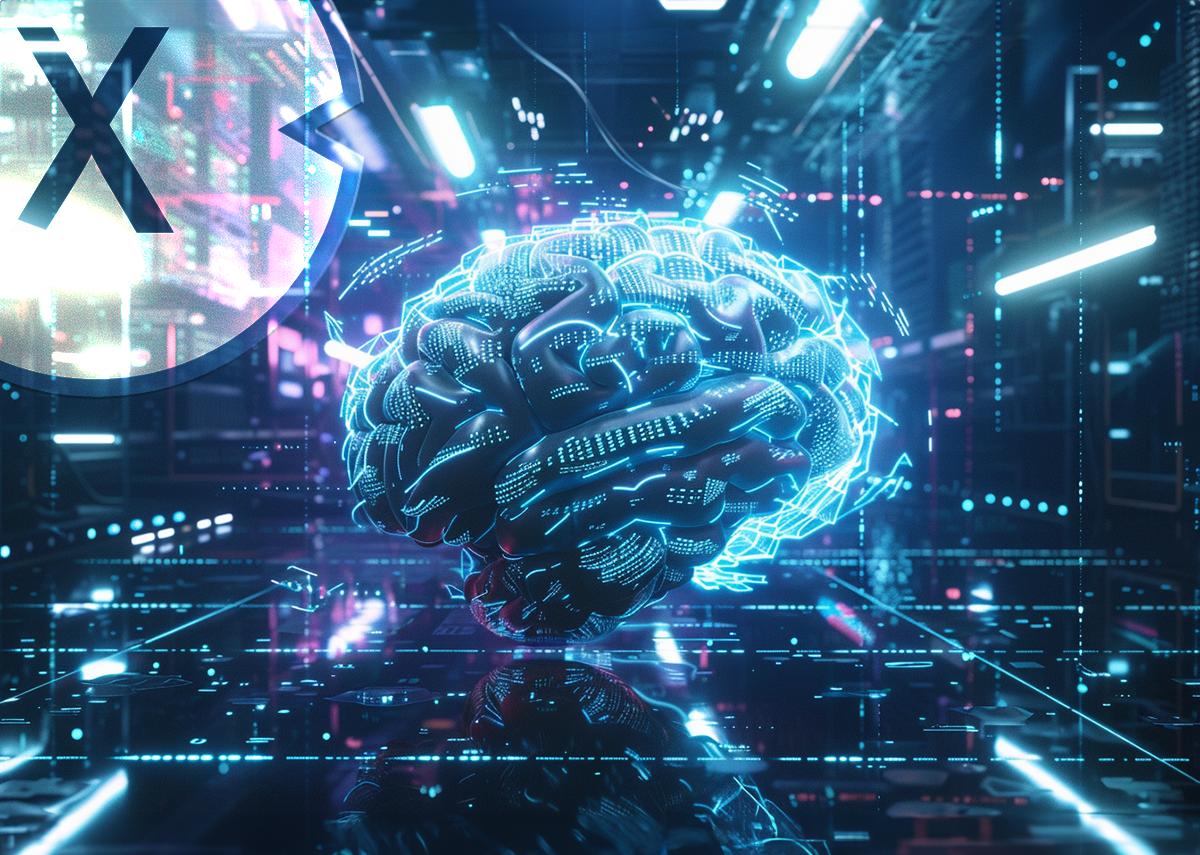
Data is the crucial component for generative AI - About the importance of data for AI - Image: Xpert.Digital
🌟🔍 Quality and diversity: Why data is essential for generative AI
🌐📊 The importance of data for generative AI
Data is the backbone of modern technology and plays a critical role in the development and operation of generative AI. Generative AI, also known as artificial intelligence, capable of creating content (such as text, images, music and even videos), is currently one of the most innovative and dynamic areas of technological development. But what makes this development possible? The answer is simple: data.
📈💡 Data: The heart of generative AI
In many ways, data is at the heart of generative AI. Without extensive amounts of high-quality data, the algorithms that power these systems would not be able to learn or evolve. The type and quality of data used to train these models largely determines their ability to produce creative and useful results.
To understand why data is so important, we need to look at the process of how generative AI systems work. These systems are trained using machine learning, particularly deep learning. Deep learning is a subset of machine learning based on artificial neural networks that mimic the way the human brain works. These networks are fed huge amounts of data from which they can recognize and learn patterns and connections.
📝📚 Text creation through generative AI: A simple example
A simple example is text creation using generative AI. If an AI is to be able to write convincing texts, it must first analyze an enormous amount of linguistic data. These data analyzes enable AI to understand and replicate the structure, grammar, semantics and stylistic devices of human language. The more diverse and extensive the data, the better the AI can understand and reproduce different linguistic styles and nuances.
🧹🏗️ Quality and preparation of data
But it's not just about the quantity of data, the quality is also crucial. High-quality data is clean, well-curated, and representative of what the AI is supposed to learn. For example, it would be unhelpful to train a text AI with data that predominantly contains faulty or incorrect information. It is equally important to ensure that the data is free of bias. Bias in the training data can cause AI to produce biased or inaccurate results, which can be problematic in many use cases, especially in sensitive areas such as healthcare or justice.
Another important aspect is the diversity of data. Generative AI benefits from a wide range of data sources. This ensures that the models are more general-purpose and able to respond to a variety of contexts and use cases. For example, when training a generative model for text production, the data should come from different genres, styles, and eras. This gives the AI the ability to understand and generate a wide variety of spellings and formats.
In addition to the importance of the data itself, the process of data preparation is also crucial. Data often needs to be processed before training the AI to maximize its usefulness. This includes tasks such as cleaning the data, removing duplicates, correcting errors, and normalizing the data. A carefully carried out data preparation process goes a long way in improving the performance of the AI model.
🖼️🖥️ Image generation through generative AI
An important area where generative AI and the importance of data is particularly evident is image generation. Techniques such as Generative Adversarial Networks (GANs) have revolutionized traditional image generation methods. GANs consist of two neural networks that compete against each other: a generator and a discriminator. The generator creates images, and the discriminator evaluates whether these images are real (from a training dataset) or generated (by the generator). Through this competition, the generator continuously improves until it can produce deceptively real images. Here too, extensive and diverse image data is necessary to enable the generator to create realistic and detailed images.
🎶🎼 Music composition and generative AI
The importance of data also extends to the realm of music. Generative music AIs use large databases of music pieces to learn the structures and patterns characteristic of particular musical styles. With this data, AIs can compose new pieces of music that are stylistically similar to the works of human composers. This opens up exciting opportunities in the music industry, for example in the development of new compositions or personalized music production.
📽️🎬 Video production and generative AI
Data also has invaluable value in video production. Generative models are able to create videos that appear realistic and are innovative. These AIs can be used to create special effects for films or to create new scenes for video games. The underlying data can consist of millions of video clips containing different scenes, perspectives and movement patterns.
🎨🖌️ Art and generative AI
Another area that benefits from generative AI and the importance of data is art. Artistic AI models create stunning works of art that are inspired by the masters of the past or introduce entirely new artistic styles. These systems are trained on datasets containing works by different artists and eras to capture a wide range of artistic styles and techniques.
🔒🌍 Ethics and data protection
Ethics also plays an important role when it comes to data and generative AI. Because the models often use large amounts of personal or sensitive data, privacy concerns must be taken into account. It is important that data is used fairly and transparently and that individuals' privacy is protected. Companies and research institutions must ensure that they handle data responsibly and that the AI systems they develop meet ethical standards.
In conclusion, data is the critical component for the development and success of generative AI. They are not only the raw material from which these systems draw their knowledge, but also the key to achieving their full potential in a variety of application areas. Through careful data collection, processing and use, we can ensure that generative AI systems are not only more powerful and flexible, but also ethical and safe. The journey of generative AI is still in its early stages, and the role of data will continue to be central.
📣 Similar topics
- 📊 The essence of data for generative AI
- 📈 Data quality and diversity: Key to AI success
- 🎨 Artificial Creativity: Generative AI in Art and Design
- 📝 Data-based text creation through generative AI
- 🎬 Revolution in video production thanks to generative AI
- 🎶 Generative AI composes: The future of music
- 🧐 Ethical considerations in the use of data for AI
- 👾 Generative Adversarial Networks: From Code to Art
- 🧠 Deep learning and the importance of high-quality data
- 🔍 The process of preparing data for generative AI
#️⃣ Hashtags: #Data #GenerativeAI #Ethics #Copywriting #Creativity
💡🤖 Interview with Prof. Reinhard Heckel about the importance of data for AI
📊💻 Data forms the basis for AI. For the training, freely accessible data from the Internet is used, which is heavily filtered.
- It is difficult to avoid distortions when training. The models therefore attempt to give balanced answers and avoid problematic terms.
- The accuracy of AI models varies depending on the application, with every detail being relevant when diagnosing diseases, among other things.
- Data protection and data portability are challenges in the medical context.
Our data is now collected everywhere on the Internet and is also used to train large language models such as ChatGPT. But how is artificial intelligence (AI) trained, how is it ensured that no distortions, so-called biases, arise in the models and how is data protection maintained? Reinhard Heckel, Professor of Machine Learning at the Technical University of Munich (TUM), provides answers to these questions. He researches large language models and imaging methods in medicine.
🔍🤖 What role does data play in training AI systems?
AI systems use data as training examples. Large Language Models like ChatGPT can only answer questions on topics that they have been trained on.
Most of the information that general language models use for training is data that is freely available on the Internet. The more training data there is for a question, the better the results. For example, if there are a lot of good texts that describe connections in mathematics for an AI that is supposed to help with math tasks, the training data will be correspondingly good. At the same time, there is currently a lot of filtering when selecting data. From the large mass of data, only the good data is collected and used for training.
📉🧠 When selecting data, how is the AI prevented from producing, for example, racist or sexist stereotypes, so-called bias?
It is very difficult to develop a method that does not fall back on classic stereotypes and is unbiased and fair. For example, if you want to prevent the results from being distorted with regard to skin color, it is relatively easy. However, if gender is also added to skin color, situations can arise in which it is no longer possible for the model to act completely unbiased with regard to skin color and gender at the same time.
Most language models therefore try to give a balanced answer to political questions, for example, and to illuminate multiple perspectives. When training based on media content, preference is given to media that meet journalistic quality criteria. In addition, when filtering data, care is taken to ensure that certain words, for example racist or sexist, are not used.
🌐📚 In some languages there is a lot of content on the Internet, in others there is significantly less. How does this affect the quality of the results?
Most of the internet is in English. This makes Large Language Models work best in English. But there is also a lot of content for the German language. However, for languages that are not so well known and for which there are not so many texts, there is less training data and the models therefore work worse.
How well language models can be used in certain languages can be easily observed because they follow so-called scaling laws. This tests whether a language model is able to predict the next word. The more training data there is, the better the model becomes. But not only does it get better, it also gets predictably better. This can be easily represented by a mathematical equation.
💉👨⚕️ How accurate does AI have to be in practice?
This depends very much on the respective area of application. For photos that are post-processed using AI, for example, it doesn't matter whether every hair is in the right place at the end. It's often enough if a picture looks good in the end. Even with Large Language Models, it is important that the questions are answered well; whether details are missing or incorrect is not always crucial. In addition to language models, I also research in the area of medical image processing. It is very important here that every detail of the image created is correct. If I use AI for diagnoses, it must be absolutely correct.
🛡️📋 The lack of data protection is often discussed in connection with AI. How is it ensured that personal data is protected, especially in a medical context?
Most medical applications use patient data that is anonymized. The real danger now is that there are situations in which conclusions can still be drawn from the data. For example, MRI or CT scans can often be used to trace age or gender. So there is some actually anonymized information in the data. Here it is important to provide patients with sufficient information.
⚠️📊 What other difficulties are there when training AI in a medical context?
A major difficulty is collecting data that reflects many different situations and scenarios. AI works best when the data it is applied to is similar to the training data. However, the data differs from hospital to hospital, for example in terms of patient composition or the equipment that generates data. There are two options to solve the problem: either we manage to improve the algorithms or we have to optimize our data so that it can be better applied to other situations.
👨🏫🔬 About the person:
Prof. Reinhard Heckel conducts research in the field of machine learning. He works on the development of algorithms and theoretical foundations for deep learning. One focus is on medical image processing. He also develops DNA data storage and is working on the use of DNA as a digital information technology.
He is also a member of the Munich Data Science Institute and the Munich Center for Machine Learning.
We are there for you - advice - planning - implementation - project management
☑️ Industry expert, here with his own Xpert.Digital Industry Hub with over 2,500 specialist articles
I would be happy to serve as your personal advisor.
You can contact me by filling out the contact form below or simply call me on +49 89 89 674 804 (Munich) .
I'm looking forward to our joint project.
Xpert.Digital - Konrad Wolfenstein
Xpert.Digital is a hub for industry with a focus on digitalization, mechanical engineering, logistics/intralogistics and photovoltaics.
With our 360° business development solution, we support well-known companies from new business to after sales.
Market intelligence, smarketing, marketing automation, content development, PR, mail campaigns, personalized social media and lead nurturing are part of our digital tools.
You can find out more at: www.xpert.digital - www.xpert.solar - www.xpert.plus