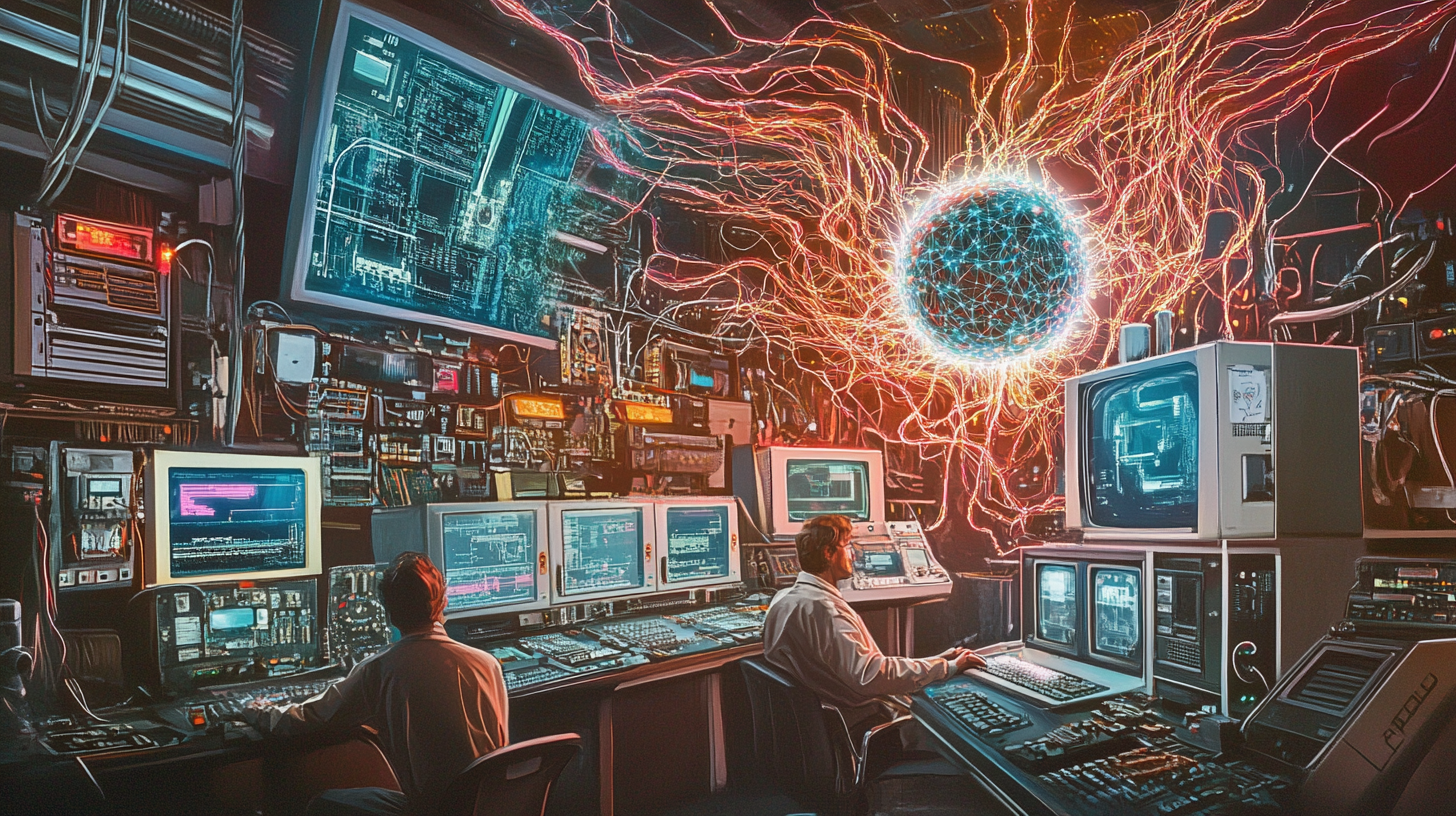
From the origins of artificial intelligence: How the 1980s laid the foundation for today's generative models - Image: Xpert.Digital
Pioneers of AI: Why the 1980s were the decade of the visionaries
Revolutionary 80s: The birth of neural networks and modern AI
The 1980s was a decade of change and innovation in the world of technology. As computers increasingly found their way into businesses and households, scientists and researchers worked to make machines more intelligent. This era laid the foundation for many of the technologies we take for granted today, particularly in the area of artificial intelligence (AI). The advances of this decade were not only groundbreaking, but have profoundly influenced how we interact with technology today.
The rebirth of neural networks
After a period of skepticism about neural networks in the 1970s, they experienced a renaissance in the 1980s. This was largely thanks to the work of John Hopfield and Geoffrey Hinton.
John Hopfield and the Hopfield Networks
In 1982, John Hopfield presented a new model of neural networks, which later became known as the Hopfield network. This network was able to store patterns and retrieve them through energetic minimization. It represented an important step towards associative memory and showed how neural networks can be used to robustly store and reconstruct information.
Geoffrey Hinton and the Boltzmann machine
Geoffrey Hinton, one of the most influential AI researchers, developed the Boltzmann machine together with Terrence Sejnowski. This stochastic neural network system could learn complex probability distributions and was used to recognize patterns in data. The Boltzmann machine laid the foundation for many later developments in the field of deep learning and generative models.
These models were groundbreaking because they showed how neural networks could be used to not only classify data, but also generate new data or complete incomplete data. This was a decisive step towards the generative models that are used in many areas today.
The rise of expert systems
The 1980s were also the decade of expert systems. These systems aimed to codify and leverage the expertise of human experts in specific domains to solve complex problems.
Definition and Application
Expert systems are based on rule-based approaches in which knowledge is stored in the form of if-then rules. They have been used in many fields including medicine, finance, manufacturing and more. A well-known example is the medical expert system MYCIN, which helped diagnose bacterial infections.
Importance for AI
Expert systems showed the potential of AI in practical applications. They demonstrated how machine knowledge can be used to make decisions and solve problems that previously required human expertise.
Despite their success, expert systems also demonstrated the limitations of rule-based approaches. They were often difficult to update and did not handle uncertainty well. This led to a rethink and created space for new approaches in machine learning.
Advances in Machine Learning
The 1980s marked a transition from rule-based systems to data-driven learning methods.
Backpropagation algorithm
A key breakthrough was the rediscovery and popularization of the backpropagation algorithm for neural networks. This algorithm made it possible to efficiently adjust the weights in a multilayer neural network by propagating the error backwards through the network. This made deeper networks more practical and laid the foundation for today's deep learning.
Simple generative models
In addition to classification tasks, researchers began to develop generative models that learned the underlying distribution of the data. The Naive Bayes classifier is an example of a simple probabilistic model that, despite its assumptions, has been successfully used in many practical applications.
These advances showed that machines not only had to rely on predefined rules but could also learn from data to complete tasks.
Technological challenges and breakthroughs
Although theoretical advances were promising, researchers faced significant practical challenges.
Limited computing power
The hardware of the 1980s was very limited compared to today's standards. Training complex models was time-consuming and often prohibitively expensive.
The vanishing gradient problem
When training deep neural networks with backpropagation, a common problem was that the gradients in the lower layers became too small to allow effective learning. This made training deeper models much more difficult.
Innovative solutions:
Restricted Boltzmann Machines (RBMs)
To address these problems, Geoffrey Hinton developed the Restricted Boltzmann Machines. RBMs are a simplified version of the Boltzmann machine with restrictions in the network structure, which made training easier. They became building blocks for deeper models and enabled layer-by-layer pre-training of neural networks.
Layered pre-training
By gradually training a network, one layer at a time, researchers were able to train deep networks more effectively. Each layer learned to transform the output of the previous layer, resulting in better overall performance.
These innovations were crucial in overcoming the technical hurdles and improving the practical applicability of neural networks.
The longevity of 80s research
Many of the deep learning techniques used today have their origins in the work of the 1980s - Image: Xpert.Digital
The concepts developed in the 1980s not only influenced research at the time, but also paved the way for future breakthroughs.
The FAW Ulm (Research Institute for Application-Oriented Knowledge Processing), the first independent institute for artificial intelligence, was founded in 1987. Companies such as DaimlerChrysler AG, Jenoptik AG, Hewlett-Packard GmbH, Robert Bosch GmbH and several others were involved. I was there as a research assistant from 1988 to 1990 .
Foundation for deep learning
Many of the deep learning techniques used today have their origins in work from the 1980s. The ideas of the backpropagation algorithm, the use of neural networks with hidden layers and layer-by-layer pre-training are central components of modern AI models.
Development of modern generative models
The early work on Boltzmann machines and RBMs influenced the development of Variational Autoencoders (VAEs) and Generative Adversarial Networks (GANs). These models make it possible to generate realistic images, text and other data and have applications in areas such as art, medicine and entertainment.
Influence on other research areas
The methods and concepts from the 1980s have also influenced other fields such as statistics, physics and neuroscience. The interdisciplinarity of this research has led to a deeper understanding of both artificial and biological systems.
Applications and effects on society
The advances of the 1980s led to specific applications that form the basis for many of today's technologies.
Speech recognition and synthesis
Early neural networks were used to recognize and reproduce speech patterns. This laid the foundation for voice assistants like Siri or Alexa.
Image and pattern recognition
The ability of neural networks to recognize complex patterns has found applications in medical imaging, facial recognition, and other security-related technologies.
Autonomous systems
The principles of machine learning and AI from the 1980s are fundamental to the development of autonomous vehicles and robots.
1980s: Intelligent learning and generation
The 1980s were undoubtedly a decade of change in AI research. Despite limited resources and numerous challenges, researchers had a vision of intelligent machines that could learn and generate.
Today we are building on these foundations and experiencing an era in which artificial intelligence is present in almost every aspect of our lives. From personalized recommendations on the Internet to breakthroughs in medicine, technologies that began in the 1980s are driving innovation.
It is fascinating to see how ideas and concepts from this time are implemented today in highly complex and powerful systems. The work of the pioneers has not only enabled technical advances, but also sparked philosophical and ethical discussions about the role of AI in our society.
The research and developments of the 1980s in the field of artificial intelligence were crucial in shaping the modern technologies we use today. By introducing and refining neural networks, overcoming technical challenges, and visioning to create machines that can learn and generate, this decade's researchers have paved the way for a future in which AI plays a central role.
The successes and challenges of this time remind us how important basic research and the pursuit of innovation are. The spirit of the 1980s lives on in every new AI development and inspires future generations to continue to push the boundaries of what is possible.
Suitable for:
- Frequently asked question, here is the answer: Artificial intelligence in the company – in-house development or ready-made solution? | AI strategy
- Understanding question on the subject of digitalization and artificial intelligence: What other AI models are there in addition to the AI language model?