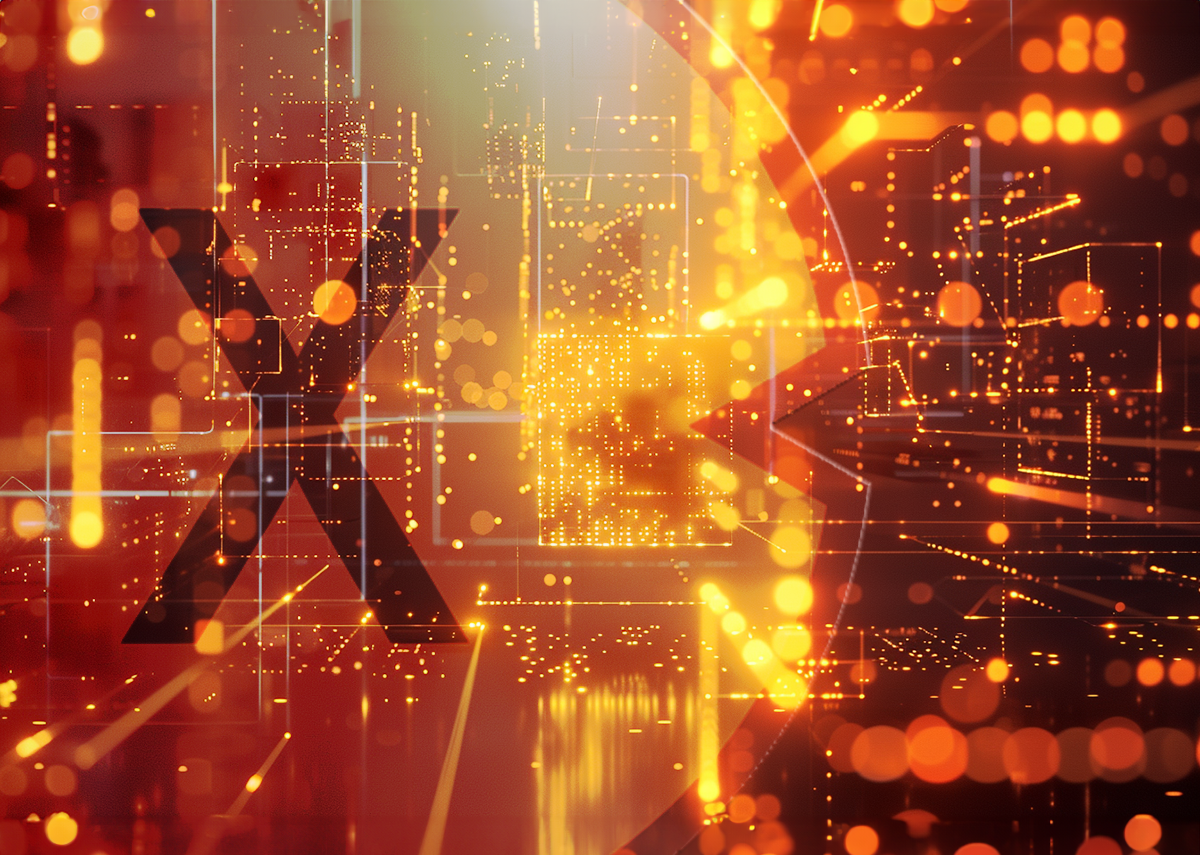
Why content AI is also a generative AI model, but not always an AI language model - Image: Xpert.Digital
🌐🔍 The versatility of AI models
🤖📄 A content AI can be a generative AI model, but not necessarily a language model. To better understand this, one must consider the distinction between discriminative and generative AI models and their respective areas of application.
Suitable for:
🧩 Discriminative vs. Generative AI models
In artificial intelligence (AI), a fundamental distinction is made between discriminative and generative models. These two approaches specialize in different types of tasks. Discriminative models aim to analyze, classify and recognize patterns in existing data. They are typically trained to make predictions or decisions based on the training data. An example is sentiment analysis, in which a model decides whether a certain text is positive, neutral or negative.
Generative models, on the other hand, have the ability to produce new data that is similar to the data they were trained on. This means that they can not only analyze or classify, but actually create something new. This ability makes them particularly valuable in areas such as text generation, image creation, or even music synthesis. A well-known example is the GPT-4 generative language model, which can generate natural language that is difficult to distinguish from human text.
📚 Language models and their role
An AI language model is a model that has been trained to understand, analyze, and process natural language. This means it can analyze, classify or translate texts. A good example here is BERT (Bidirectional Encoder Representations from Transformers), a discriminative model that analyzes text without generating new data. It recognizes the context and meaning of words within a sentence and can take on tasks such as question answering or text classification.
However, not every language model is generative. Some models are purely discriminative and focus on understanding and analyzing texts. They are optimized to recognize patterns in input data to make predictions or perform specific tasks, such as detecting fake news or identifying spam emails.
🔗 The connection between language models and generative models
Language models can also be generative models. However, this depends on their construction and purpose. A generative language model is capable of creating new text that is similar to the training data. It uses statistical patterns learned during training to generate plausible text sequences. A particularly powerful generative model is GPT-4, which has been trained with billions of parameters and is capable of composing human-like text by imitating the structures and patterns in human language.
GPT-4 uses the Transformer architecture, which has proven particularly effective for language models in recent years. The Transformer is based on a mechanic called Self-Attention, which allows the model to understand the context of a word within a sentence or a longer text and thus determine the next logical step. This ability makes GPT-4 particularly good at generating text that is coherent and grammatically correct.
📊 Market shares and distribution
The market for AI models is broad, and there are numerous vendors and open source projects that provide both discriminative and generative models. OpenAI, the company behind GPT-4, is one of the leading developers of generative AI models. GPT-4 is used in various industries, from content creation to automating customer service interactions to medical research, where it helps analyze and generate research reports.
On the other hand, there are companies like Google with its BERT model, which has a significant impact on the field of discriminative AI models. While generative models are becoming increasingly important, particularly in the area of content creation, discriminative models continue to play a crucial role in areas where the analysis and interpretation of data is paramount.
📝 Applications of generative language models
Generative language models are used in many areas. Some of the most notable use cases are:
1. Copywriting
Generative language models can automatically compose texts such as news articles, reports, emails or even creative literature. Such models are used in the content marketing industry to automatically create content for blogs, social media and websites.
2. Customer Support
Chatbots and virtual assistants use generative language models to provide natural and fluid answers to customer queries. This not only improves efficiency, but also customer satisfaction as answers can be provided faster and more accurately.
3. Translation
Some generative language models are trained to translate texts from one language to another by generating new sentences in the target language that preserve the semantic content of the original text. Such models enable translations that better reflect the nuances of human language.
4. Image generation with text
In combination with other generative models, language models such as DALL·E can generate images from text descriptions. This opens up whole new possibilities in the advertising and design industry as custom visual content can be created simply by entering text.
🚀 Future developments and challenges
Although generative language models like GPT-4 produce impressive results, there are still challenges. One of them is controlling the output quality. Generative models sometimes cannot provide the desired information content or accuracy because they are based on probabilities and do not always fully understand what they generate.
Another problem is the bias in the models. Because generative models rely on large amounts of training data sourced from the Internet, they can inadvertently inherit biases and stereotypes present in the data. Companies and research institutions are continually working to minimize these problems by refining training processes and implementing specialized filters.
Bias in AI models refers to the distortions or prejudices that come from the training data. Because generative models are often trained on large datasets obtained from the Internet, this data can contain biases and stereotypes. These biases can be unintentionally incorporated into the models, leading to biased results. Researchers and companies are working to minimize these biases by refining training processes and implementing specialized filters.
For example, Amazon had to switch off its AI for evaluating applicants because the automatic evaluation system disadvantaged women .
🛠️ Strengths and areas of application
Generative and discriminative AI models both have their specific strengths and areas of application. Language models play a central role here, as they can be used in various industries for a variety of tasks. While generative language models are capable of producing creative and human-like text, discriminative models remain an indispensable tool for analyzing and processing existing data.
In summary it can be said that:
- A language model does not always have to be a generative model. Many language models specialize in understanding and analyzing existing data without generating new data.
- Generative language models, on the other hand, can generate new text and are therefore often used in areas where creativity and innovation are required.
- The future of AI will likely see increased integration of generative and discriminative models to create even more versatile and powerful systems.
This development will further increase the impact of AI on various industries, from automating simple tasks to assisting with complex, creative processes.
Suitable for:
📣 Similar topics
- 🤖 Different AI models at a glance
- 📊 Discriminative vs. Generative AI Models: A Comparison
- 📈 The applications of generative language models
- 🧠 How GPT-4 imitates human speech
- 🖼️ Image generation through text: The power of generative models
- 💡 Areas of application of language-based AI models
- 🌐 Market shares and distribution of AI models
- 🔄 The future of integrating discriminative and generative AI models
- 💬 The role of language models in AI
- ⚖️ Challenges and biases in generative models
#️⃣ Hashtags: #GenerativeKI #DiscriminativeKI #Language Models #GPT4 #AIApplications
We are there for you - advice - planning - implementation - project management
☑️ SME support in strategy, consulting, planning and implementation
☑️ Creation or realignment of the digital strategy and digitalization
☑️ Expansion and optimization of international sales processes
☑️ Global & Digital B2B trading platforms
☑️ Pioneer Business Development
I would be happy to serve as your personal advisor.
You can contact me by filling out the contact form below or simply call me on +49 89 89 674 804 (Munich) .
I'm looking forward to our joint project.
Xpert.Digital - Konrad Wolfenstein
Xpert.Digital is a hub for industry with a focus on digitalization, mechanical engineering, logistics/intralogistics and photovoltaics.
With our 360° business development solution, we support well-known companies from new business to after sales.
Market intelligence, smarketing, marketing automation, content development, PR, mail campaigns, personalized social media and lead nurturing are part of our digital tools.
You can find out more at: www.xpert.digital - www.xpert.solar - www.xpert.plus